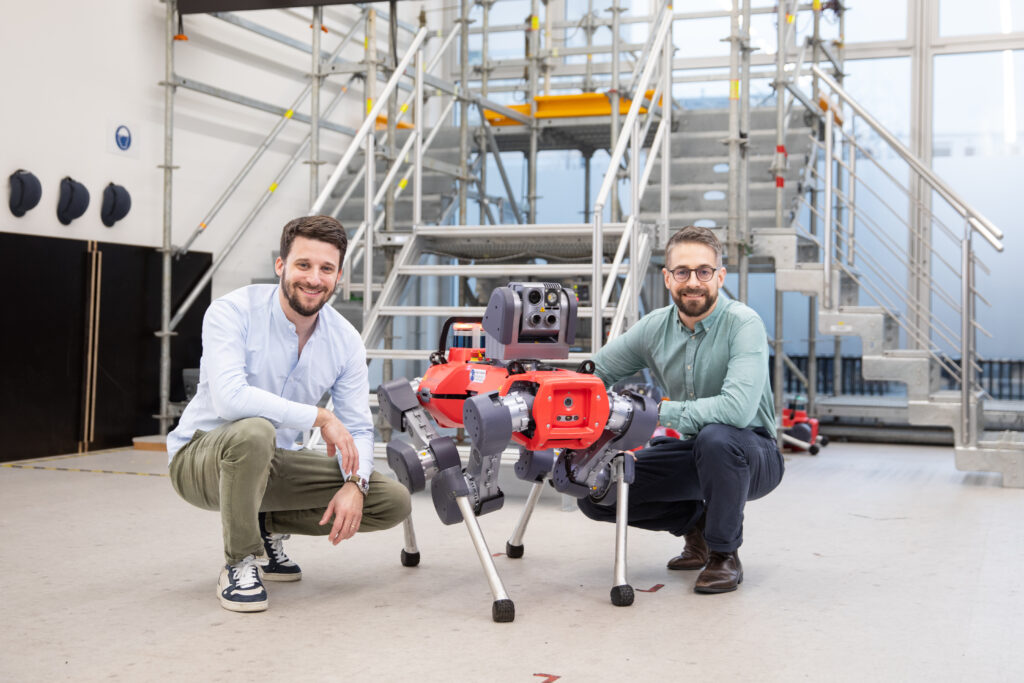
Anthropic is an AI powerhouse built to prioritize safety and responsibility. It’s not set up as a traditional company; instead, it’s a public benefit corporation, which means that its board has both a traditional fiduciary duty to earn money for shareholders and a mission to ensure the company operates in a way that “helps people and society flourish.”
Claude, the company’s powerful LLMs, put Anthropic at the forefront of the AI industry.
Matt Bell leads product research for Anthropic and joined Qualcomm Ventures’ 15th CEO Summit to talk about the state of AI and his team’s work to build the capabilities and increase ease of use for Anthropic’s Claude models for enterprises. An accomplished entrepreneur and engineer, he previously founded Matterport, a leading spatial computing platform. At the Qualcomm Ventures CEO Summit, Matt sat down for a fireside chat with Albert Wang from Qualcomm Ventures whose investment areas include enterprise software, AI, data, cloud platforms, and internet of things. This interview is adapted from their conversation.
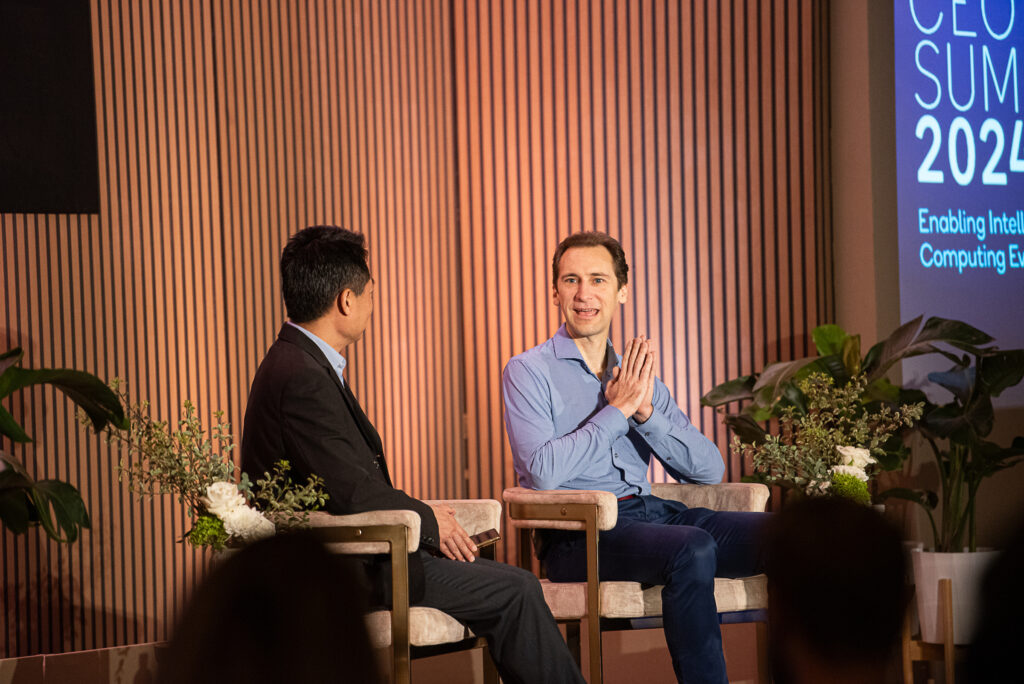
Albert Wang: Thanks for joining us today, Matt. Let’s start with the company’s name. What’s the meaning behind Anthropic? And how does it fit with the company’s long-term vision and approach?
Matt Bell: Thanks for having me. So, the word anthropic means “relating to humanity.” We chose it as the name for the company because we wanted to emphasize that we’re putting humans at the center of our technology, and also to reflect our emphasis on human feedback and human interaction.
Also, Anthropic is a public benefit corporation. Profit is important of course, but it is not our only motive. We believe AI should be to the benefit of all of humanity. For example, we devote a large fraction of our company’s resources to AI safety research — not just near-term questions of trust and safety, but also in terms of thinking about how we ensure AI remains aligned with benefits to humanity as we approach AGI. And we have collaborations with third parties to think through the societal impacts of AI and ensure that AI is developed with universal human principles in mind.
I should also note that Anthropic is governed by a Long-Term Benefit Trust, which has the authority to select members of our board of directors. This structure is designed to align our corporate governance with our mission for developing and maintaining advanced AI for the long-term benefit of humanity. Members of the trust include Neil Buddy Shah, CEO of the Clinton Health Access Initiative; Kanika Bahl, CEO and president of Evidence Action, which works to address global poverty; and Zach Robinson, interim CEO of Effective Ventures US, which helps to support important organizations working for positive change around the world. In other words, these are people from the nonprofit sector who are used to thinking about large-scale human flourishing.
AW: As you say, Anthropic has a big focus on research. Given that, what frontiers do you plan to explore and push forward in AI technology? Multimodal, reasoning, agentic AI, spatial intelligence? What could bring us closer to AGI?
MB: To start, we’re really focused at Anthropic on what we call, “just do the basic thing well.” Claude is our AI assistant, and it’s trained to be safe, accurate, and secure. In the last year, we’ve spent a lot of time on things like getting Claude’s hallucination rate very low; getting Claude to remember facts across its entire 200k context, write code that compiles, follow the instructions in the system prompt, and use tools reliably even when there are a lot of tools to choose from; and also improving both Claude’s ability to index and search knowledge bases and its basic perception and reading ability.
It’s the “boring” things like this that will make these models successful and enable them to deliver so much value to business users and consumers alike. And I think doing all of those things well, and with even more reliability, is what will get us closer to AGI.
That said, I’m excited by agents, and I know a lot of other people are as well. Commercial agent deployments tend to look more like workflows — that is, a system of prompts that process and route information. But there’s also a lot of experimentation with true agents — agents that have a self-reflection loop and can take an arbitrary series of actions. Doing that well requires us to solve problems like long-horizon planning, self-reflection, and self-correction. And we’ve seen a lot of progress over the last few months on agent benchmarks and evaluation tools like SWE-bench.
Also, we’re very excited for automated prompt engineering. There’s often a big gap between what models are capable of doing and how they’re actually being used, often due to the trickiness of getting a good prompt. We released a prompt generator that turns a user’s idea into a prompt incorporating all of our best practices. That’s making a big difference.
AW: As a leading foundational model, what do you see as the current stage of enterprise adoption of Gen AI applications? How much is still experimentation, as opposed to actual commercial production?
MB: There is actually already a lot of scaled commercial deployment, in many use cases — including customer support chatbots, backend and workflow automation, coding assistants, and so on. There are also AI-native startups like Perplexity, Cursor, and Cognition, where their whole product is built around Claude. We’re also seeing enterprise SaaS companies like Salesforce, Notion, Vercel, and many others across nearly every industry globally using Claude to turbocharge their products and provide natural language interfaces for their underlying complexity. And there are also large industry players like Intuit that have added AI to mature products.
So it’s definitely not just experimentation at this point.
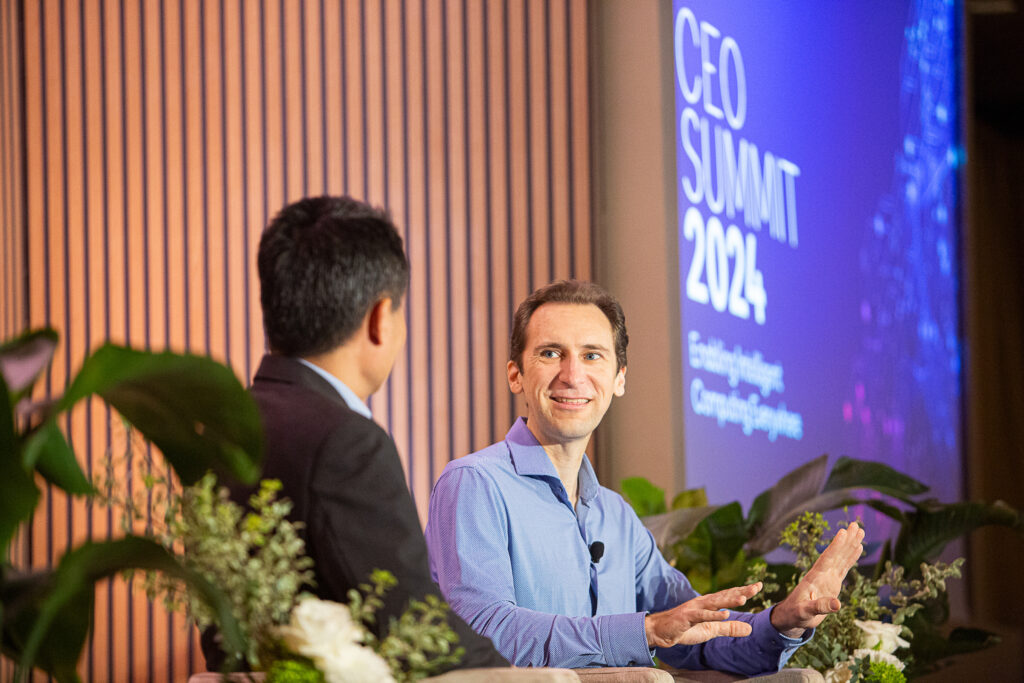
AW: For enterprises that haven’t been able to successfully customize and implement Gen AI models, what do you see as the bottleneck? Hallucination? Fine-tuning/RAG know how? Prompt engineering?
MB: Working with LLMs often requires a significant change in thinking from standard software development practices. For example, prompts are a lot “squishier” than code, so you have to approach prompt construction more like instructing an employee than like programming a computer. As a result, customers need to invest heavily in learning how to prompt as well as in writing comprehensive evals. That can be a bottleneck, and we’ve spent a lot of time developing tools to address it.
Integration of knowledge is also often a challenge. Retrieval-automated generation, or RAG, was touted as a cure for this, but I joke that RAG is like putting your docs through a shredder before handing them to the model. We’ve come up with some superior alternatives at Anthropic, such as contextual retrieval and prompt-caching of long contexts.
Also, hallucinations and jailbreaks used to be a big problem, but better models have reduced both, and techniques like guardrails can further reduce jailbreaks.
AW: Deep learning based AI technology today is inductive reasoning by nature and inherently prone to hallucination. Do you see possible paths for AI to adopt deductive reasoning and produce more reliable outcomes?
MB: One possibility I see is to emulate deductive reasoning using inductive reasoning. Humans have been doing that successfully for hundreds of years despite the inductive reasoning nature of human brains.
AW: What’s Anthropic’s view on bringing Gen AI to edge devices like smartphones, laptops, and cars?
MB: Edge device networks make the most sense for high-bandwidth-sensing, low-cost-per-token, low-latency applications. Augmented reality, navigation, and robotics all make sense to do at the edge. Anything with a live video feed is ideal for it, as Qualcomm Technologies, Inc. has recognized. I think we’ll likely see hybrid models, where the local model handles everything moment-to-moment but talks to the cloud model for things like longer-term planning.
AW: Now that the genie is out of the bottle, what are you worried about that could go wrong with AI? And what is Anthropic doing to address the related safety issues?
MB: Short-term issues that worry me broadly – not specific to Anthropic – are trust and safety violations like spam, harassment, impersonation, hate speech, election interference, and so on. Unfettered AI allows bad actors to take these kinds of bad-faith actions at scale. The longer-term issue is that uncontrolled powerful AI systems could be a catastrophic risk for humanity. And that’s something Anthropic takes very seriously. We think the benefits of powerful AI outweigh the risks, but at the same time we only proceed if we think it’s safe.
We have a Responsible Scaling Policy in place that creates capabilities gates, meaning we will not train models that exceed a certain level of capability, whether in terms of potential harms or autonomy, until certain safety measures are met. That policy is public, and it covers the various kinds of risks we’re concerned about, including deployment risks — in other words, what could a malicious user do? — and containment risks — meaning, if the model weights are stolen, what could a malicious entity do, given free rein to fine-tune or change the model?
AW: Final question. What’s Anthropic’s take: open source or proprietary foundation models? Race to the bottom or race to the top?
MB: I think smaller, open-source models can be great. They are lightweight, they can run locally, and they can be adapted for a lot of uses. They give maximum likelihood estimation frameworks, or MLEs, a chance to build their skills.
I’m more concerned about frontier open-source models. People creating those can put all the safety fine tuning they want, but that’s easily reversible by someone who knows what they’re doing. As a result, open-source models can be abused by bad actors to create spam, spread disinformation, harass people, and so on. This is bad, and the downsides will get worse as models get more powerful. You could imagine a hostile state or non-state actor using an advanced open-source model to do bioweapons research or launch a massive cyberattack.
That’s why we encourage a race to the top via the responsible scaling policy. And we want other frontier model providers to adopt similar approaches.
To learn more about Anthropic, visit here.
All opinions expressed are solely those of Matt Bell.